What is AI's Job with a SIEM?
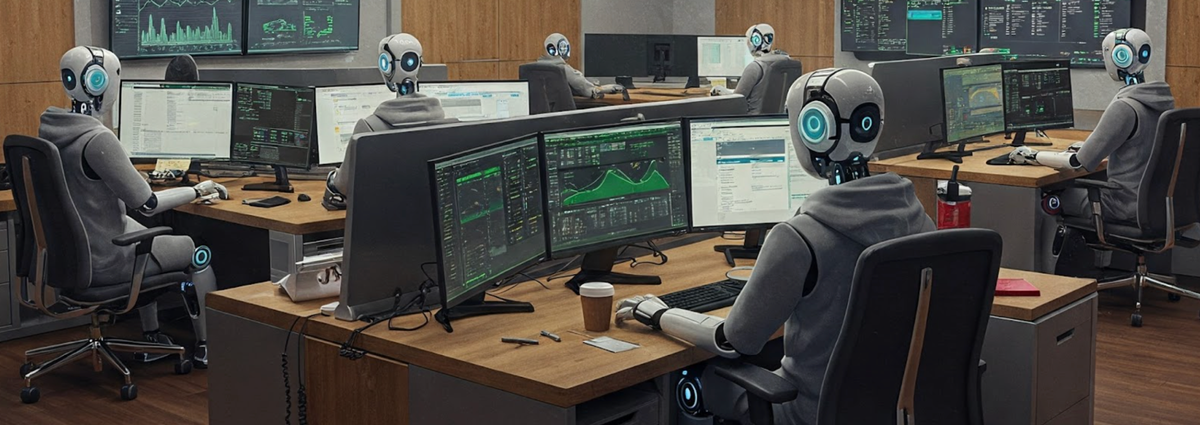
The rise of generative AI has reignited excitement across cybersecurity, with many looking to these tools as accelerants for investigation. The most immediate applications focus on assisting analysts—helping them write better queries, summarize data, or navigate the SIEM interface with natural language. These use cases feel intuitive, especially in systems built around query-driven workflows. But this approach also reflects a deeper architectural limitation: it assumes that the primary task of a SIEM is still a search.
In most cases today, AI is not helping solve detection—it’s helping people look things up. That’s not a transformative shift. It’s a convenience layer for an older model.
To understand where AI can truly reshape operations, we need to return to the event hierarchy: raw events at the base, followed by notable, critical, and then correlated cases. Most early AI work sits at the bottom half of that structure—trying to classify or explain atomic or critical events one at a time. But as anyone who has dealt with alert overload knows, analyzing each event individually—even with AI assistance—still breaks at scale.
Generative AI isn’t immune to scale. You pay by the token, and models are limited by context size. Trying to run every alert through LLMs is cost-prohibitive and often redundant. The signal-to-noise problem doesn’t vanish just because the interpreter is smarter. If anything, it becomes more expensive.
This is why Fluency’s architecture places traditional AI/ML—pattern recognition, clustering, and deviation detection—in the stream. By doing that, we reduce millions of raw events to a much smaller set of meaningful clusters. These correlated groupings—typically fewer than 30 a day—are where generative AI can add real value. Not by parsing endless noise, but by interpreting what’s already been distilled: helping analysts reason about those clusters, propose next actions, or even summarize the evidence for communication or ticketing. It’s AI as an assistant, not AI as a search engine.
The key insight here is that generative AI works best downstream of intelligent selection. The smarter the SIEM, the less work the AI has to do—and the more strategic its contribution becomes. In systems that fail to reduce the data meaningfully, generative AI becomes a patch for bad architecture. In systems that front-load analysis and preserve state, AI becomes an amplifier.
And this doesn’t just affect cost. It affects readiness. AI can write reports, but it can also trigger workflows. Fluency’s direction includes not just analysis but decision-making support: moving from query assistant to response co-pilot. This is only possible because the system already understands the difference between raw data and a real case. It doesn’t treat every event like a mystery to be solved. It treats each cluster as a hypothesis to be tested, validated, and explained.
Finally, we need to recognize a subtle but important point: many SIEMs today still focus on visual dashboards—charts meant to help humans make sense of data. But charts are for people. AI doesn’t need bar graphs or donut wheels to understand a pattern. It needs a structured state, temporal relationships, and event context. The more the system builds those layers natively, the less “interpreting” the AI has to do.
So while many vendors pursue AI as a bolt-on—another feature to market—the real opportunity is foundational. The better your pipeline, the more meaningful your data structures, the clearer your detection logic, and therefore the more value you’ll get from AI. Not because AI is magic—but because we have made the problem solvable.