AI Is Turning Security Operations Inside Out
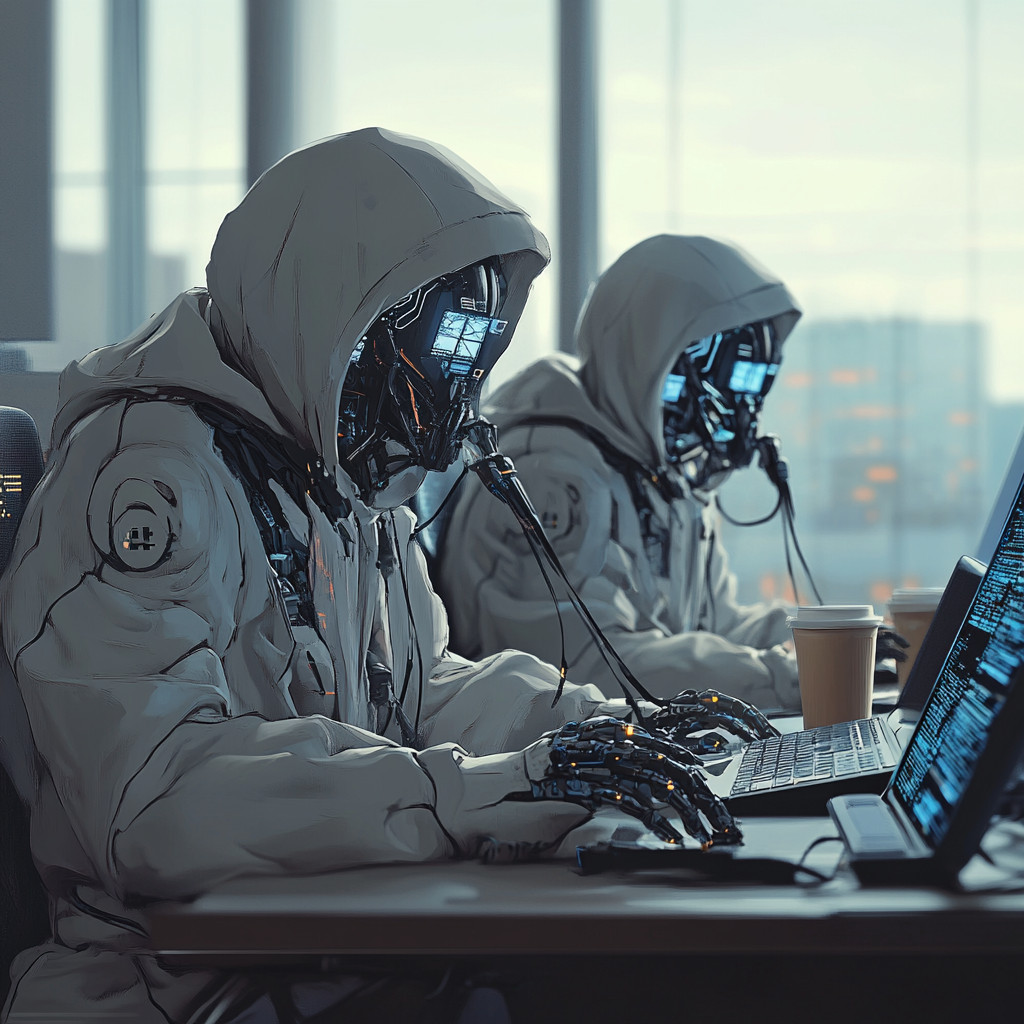
Security operations have always faced the fundamental challenge of scale. Years ago, when I started working in cybersecurity, we knew the volume of data would eventually overwhelm human analysis. That moment has arrived, and AI is now forcing a complete rethinking of how security operations centers function.
What's happening isn't just automation—it's a fundamental shift in how we approach security. The traditional three-tiered SOC model is being inverted, with AI taking over first-tier analysis functions at a level that exceeds what human analysts can achieve. This isn't about replacing humans; it's about redefining their roles in the security ecosystem.
AI Excels Where Humans Struggle
The most immediate impact of AI is addressing the scalability problem. When millions—sometimes billions—of security events flow through systems daily, human analysts simply cannot keep pace. AI allows for well-reasoned analysis across this massive volume of data.
But there's a critical distinction many security leaders miss: AI isn't just one thing. When most people think of AI in security, they immediately jump to large language models (LLMs) like ChatGPT. These models get attention but represent just one approach.
At Fluency, we've developed statistical clustering techniques and fault tolerance analysis methods that prove far more effective for analyzing security events at scale. These specialized models work better for high-volume data processing than general-purpose LLMs, which are comparatively slow for real-time security operations.
This modular approach mirrors how our own brains function. The human brain isn't a single processing system but different regions handling specialized tasks. Security AI needs multiple models working in concert—each optimized for specific security functions.
Current SIEMs Hobble AI's Potential
Traditional SIEM architectures fundamentally limit what AI can accomplish. These systems present data "atomically"—giving small, disconnected pieces and expecting analysts to assemble the puzzle. That approach made sense when humans were doing all the analysis, but it hamstrings AI capabilities.
It's like trying to teach someone chess by only explaining individual piece movements without showing complete games. For AI to reach its potential in security operations, we need to feed it holistic data patterns—complete "games" with beginnings, middles, and ends.
Current SIEMs not only fail to address alert fatigue; they actively hinder AI by forcing it to operate at the lowest possible level of intelligence. The architecture itself needs reimagining.
Human Analysts Will Shift, Not Disappear
As AI takes over first-tier analysis, security professionals won't become obsolete—they'll elevate to higher-value work. The human mind excels at finding anomalies, making connections between seemingly unrelated events, and bringing contextual understanding that AI currently lacks.
The truth is, attacks themselves are anomalies. AI excels at identifying patterns and normalcy, but human analysts still outperform machines at evaluating and understanding genuine anomalies—precisely what security threats represent.
This shift means security teams will move from alert management to strategic threat intelligence. SOC personnel will step back from the grunt work nobody wants to do anyways, focusing instead on the complex puzzle-solving aspects of security that humans naturally excel at.
AI wil eventually impact 2nd Tier Analysis
AI is already helping in two crucial ways: translating technical security data into understandable summaries and providing contextual enrichment across longer timeframes. The next frontier is "scoping"—finding elements from a detected attack that might reveal additional compromise elsewhere in the environment.
This represents level-two analysis in traditional SOC environments, an area where AI has yet to fully penetrate but inevitably will. The opportunity for AI to excel here is immense, provided we redesign our data collection approaches to support these higher-level functions.
The Validation Challenge
AI integration creates an unexpected vulnerability: over-reliance on AI-generated analysis without proper validation. AI excels at pattern recognition but doesn't truly "reason"—it predicts the next most likely step based on training data.
Think of AI like muscle memory in video games. The muscle isn't thinking; it's executing patterns it's seen before. We need validation mechanisms above AI to verify its conclusions, creating what amounts to a new management layer in security operations.
It's similar to the difference between a baker in a shop versus the person managing the bakery. They're distinct roles, and this oversight function becomes increasingly important as AI handles more security tasks.
The Security Training Paradox
We face a profound paradox in security workforce development. How do you train someone to oversee AI security systems when they've never performed the fundamental work the AI is now handling?
It's like expecting someone to use AI for coding when they've never coded themselves, or asking students to learn calculus while using calculators for basic arithmetic. Understanding the fundamentals remains essential for effective oversight.
During this transition period, organizations need to attract security professionals who gained fundamental experience elsewhere. Long-term, we need new mechanisms for teaching security basics—perhaps through structured validation roles where entry-level personnel verify AI conclusions.
This resembles old-world apprenticeships but presents a challenge: in traditional apprenticeships, even basic work provided business value. When AI can already perform these tasks more efficiently, how do we justify the investment in training?
Working On The System, Not In It
Looking ahead, the most successful security operations centers will shift from having people work "in the system" to having them work "on the system." Instead of humans executing security processes, they'll design, refine, and oversee the processes themselves.
Security professionals won't write code-based playbooks but will articulate security logic in natural language for AI to implement. The ability to clearly explain security concepts—what we might call "security prompt engineering"—will become immensely valuable.
The fundamental shift is from placing humans inside processes to designing processes that AI executes under human supervision. This requires a complete rethinking of security operations from the ground up.
Organizations clinging to traditional models where humans perform first-level analysis will find themselves overwhelmed by both the volume of threats and the efficiency gap between their operations and competitors using AI-enabled security approaches.
The transformation is already underway. The question isn't whether AI will reshape security operations—it's whether your organization will lead or follow in this inevitable evolution.